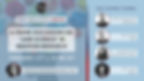
The lack of understanding of what "emotion" is has become apparent as business interest in understanding and measuring consumer emotions to improve products and services grow. While it may seem easy at first to identify basic emotions, like happiness, sadness, and anger, defining the nuances of feelings and mood spaces can be a significant challenge, as the term "emotion" means different things to different people. But the problem becomes even more obvious when trying to measure emotional changes.
Many of the issues plaguing emotion research can be attributed to a misunderstanding of the science and a reliance on researchers who are pressured to push the limits of what tools and technologies can measure. To satisfy expectations and keep the topic flashy, emotion research often falls short, with problems ranging from misused, overgeneralized tools to overreaching and unsupported claims and interpretations from those measures.
Recently, HCD Research hosted a webinar bringing together an expert panel of emotion researchers including Pedro Almeida, PhD from MindProber, Toby Coates from MMR, Michelle (Lani) Shiota, PhD from Arizona State University, and Tian Yu, PhD from Aigora to discuss the challenges of conducting sound emotion research.
This webinar aimed to shed light on these issues and provide guidance for conducting impactful emotion research. Keep reading to hear some highlights from the webinar and learn how to avoid the pitfalls of “Junk Science” in emotion research.
Let’s try to define “emotion.”
To kick this conversation off, HCD’s Michelle Niedziela, PhD prompted the panelists to try to explain exactly what is an emotion. Michelle (Lani) Shiota, PhD caveats the question by explaining how the word “emotion” is an umbrella term for psychological and biological experiences that are sometimes associated with behavior. She defines emotion as “a moderately instinctive response to what we believe is happening in the environment, and the implications of what is happening to our environment for our own well-being.” In an attempt to capture this vague, multifaceted phenomenon, researchers use tools and technology to act as imperfect indicators and help us better understand the motivation for such reactions.
Why is it so easy to make a mistake when looking into emotions? Because psychological constructs are NOT real, concrete things that can be measured without error
Pedro Almeida, PhD acknowledges a lot of emotion research is motivated by clients who are interested in proving the quality of their product and may use several approaches to find one that supports the narrative they want. The emotion measures- from self-reported responses to fMRI- are not the problem; it is the assumptions that are made about the measures. Changes measured are not equivalent to the construct being measured but serve as an indicator or proxy because of the correlations validated in research. Sweat gland activity strongly correlates with arousal, but it is a much looser correlation when the construct of arousal is stretched to something like fear or lying. Complicated emotional experiences involve context and nuance and make it challenging, sometimes impossible, to attribute the same correlations to specific scenarios.
Let’s talk about implicit association
A big distinction came about during the conversation surrounding the topic of implicit association testing. Lani addresses how often people assume it is a measure of emotion when, in fact, this test reveals concept associations, or the extent people associate a target concept word with a concept. This reaction is based on a cultural network of knowledge, which means it may not be equivalent to how we feel. Implicit testing focuses on associations between two concepts, yet the affective response about that association requires different measures, such as self-reported responses. But, Michelle calls out that this is why it is important to remember that one tool alone will never tell the whole story.
The importance of data quality
Tian Yu, PhD emphasizes how crucial it is to use the tool to collect the right type of data by asking, “Is the data enough to support the question you are asking?” She goes on to share that the model can only predict what the data has seen.
Conversations around Emotion AI reveal that clients often push researchers to use tools for the sake of using them or want to generalize and extend the data to mean something untrue about emotion. Toby Coates plays devil’s advocate by empathizing with clients who are enticed to use certain interfaces that make it seem simple.
“The truth is that there is nothing wrong with technology… [Facial coding] is not measuring emotion at all, it is measuring small facial movements.” Toby goes on to mention how context is lost in only focusing on the face rather than accounting for things like body language.
When have you gone too far?
Pedro shares that the best way to avoid over interpreting the data is to develop a hypothesis and a pre-analysis plan prior to data collection. “We try to do things hypothesis based. Let’s build this set of hypotheses, and those are the things that we will be looking at the data on. We then need multiple instances of a given event… if you have so many events, you can average out everything else that is happening. This is a really hard thing to do.”
Educating clients about the proper way to do research can be challenging, but there is a space where academics and industry leaders can work together to explore these mechanisms. The most effective partnerships come from defining a good question and setting good boundaries together.
The future of emotion research
So, what does the future hold? Here are some thoughts about opportunities in emotion research:
“Activation of measures in real-time, processing data with validity, and having outcomes of value. I also believe the [neuromarketing] industry will be scrutinized and use academic consultants to make the research better.”- Pedro
“Think about how these measures correlate with behavior rather than trying to use them to understand what is going on in people’s minds.” – Toby
“If we see the right form of the data and different factors that could affect helping these measures, we move forward on interpreting this measure to the right emotion”- Tian
“We need to do research that integrates and acknowledges that when we talk about “emotion,” we are talking about loosely correlated phenomena that appear to have some type of motivational direction. Get out of the competitive space and understand better how that system works from the strengths that we have learned, and learn applications of behavior.” - Lani
If you are interested in learning more about emotion research, please watch register for the recording here or contact Allison Gutkowski at allison.gutkowski@hcdi.net for any other inquiries.